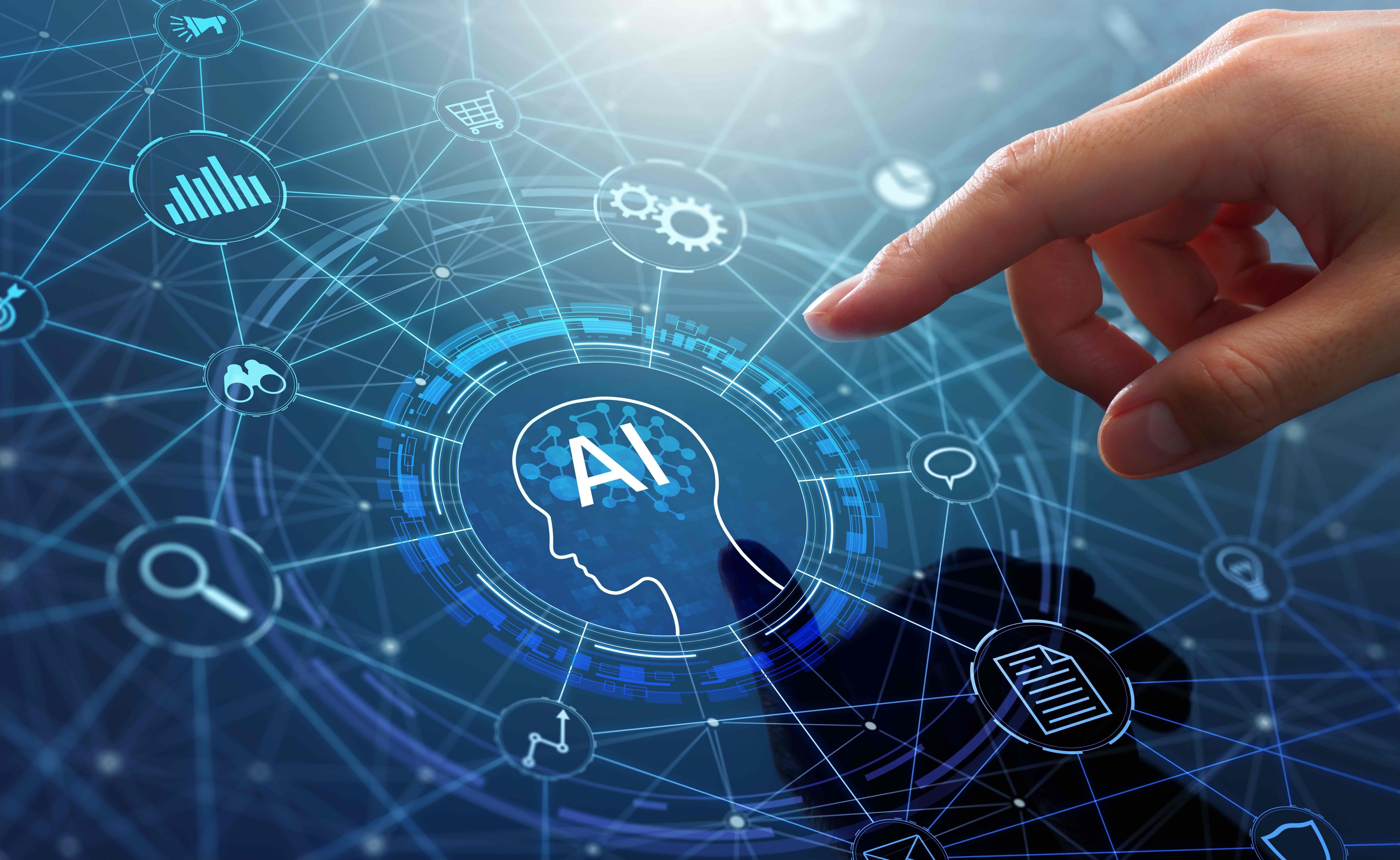
If you are new to the agentic way of problem-solving, then you might be wondering how it differs from writing code the usual way. The focus while writing code is on logic, efficiency and keeping it well-structured. The output is predictable and deterministic. While working with agents, the priority shifts to ensuring that your agent understands what you want it to do. If the intent is clear, most of the problem is solved then and there.
Revisiting most important CrewAI concepts
Before we dive into how we can improve our results while using CrewAI, let us revisit the most important concepts first.
Agent 🤖 → a unit that can perform specialized tasks, make decisions, and delegate its work to other suitable agents. Each agent has a role, goal, and backstory that describes what it is supposed to do.
Task 📋 → It contains information about what the agent should do, such as a description and expected output.
Crew 🚀 → A group of agents that work together to complete tasks.
Tool 🔧 → A helper that enhances the agent’s capabilities.
With that being said, given below are ten tips and tricks that will help drastically improve the quality of output given by your CrewAI:
1. One agent, one role
Try to make your agents more granular and as specific as possible. In the CrewAI docs, it is mentioned that for easy modeling we can imagine each agent as an actual person with a role / title who is specialized in a certain task.
This is super important in getting the best output, as agents are designed to be specialized in a particular task. By keeping that in mind, we can model our agents in the best way possible.
Let’s understand this with an example:
Suppose we are managing a blog site that publishes content regularly on trending topics. Think about how different specialized people would work on different tasks.
Content Researcher 🔍 → Identifies relevant and trending topics to write about.
Content Creator ✍️ → Writes the draft of the blog based on research and the topic selected by the Content Researcher.
Editor ✂️ → Checks for flow and grammar, improves readability, and ensures the content is SEO optimized
Here each agent performs its own task and it does that specific task well. More granular and specialized agents ⇒ Better results 🏆
2. Understand the difference between role, goal, and backstory
While defining an agent, we have to provide a role, goal, and backstory. It is essential to keep these parameters crystal clear, as they give the agent clarity on what it has to do. The next time you are writing these, ask yourself the following questions:
Role 👤 ⇾ If the agent was an actual human working on this task, what role would you give?
Goal 🎯 ⇾ What tasks is the agent expected to perform?
Backstory 📜 ⇾ What details can help the agent better fit into the role you’re assigning?
Here is one simple example:
writer = Agent(
role='Content Writer',
goal='Craft engaging blog posts about the AI industry',
backstory='A skilled writer with a passion for technology.',
verbose=True
)
3. Make the best use of tools
CrewAI provides us a set of tools, which can be used to enhance the capabilities of the agents. By using tools, we can process the data we are given and provide it in a format that the agent can understand easily.
For example, we can use the File Read Tool that allows the agent to read files present in a specific directory.
Using CrewAI, we can also write custom tools. If the problem we are trying to solve requires domain-specific operations, we can write our own tools that suit the purpose.
In general, using tools can help us process and provide the required information to the agent.
4. Provide the agent exactly the information it needs, in a way it can easily understand
Agents perform best when given clean and well-structured input. If that requires pre-processing the data beforehand, it’s worth the effort.
5. Let the agent handle complex decisions while you optimize the simple tasks
I’ll explain this one with an example. Suppose you need a list of products from the best-selling categories in a store, where the top categories are identified based on specific factors. Here we can split the work to be done as two steps:
Give the agent list of categories as input and ask it to give the best-selling categories based on the given factors
Get the products that belong to the best-selling categories
The key here is to decide which tasks should be handled by the agent and which ones we can efficiently perform ourselves. The agent’s primary role is to apply logic and decision-making 🧠 — such as identifying the best-selling categories. Fetching products that belong to best-selling categories is a straightforward filtering operation that can be done by us easily.
Instead of making the agent do everything, we optimize efficiency by letting it focus on reasoning while we handle simpler tasks programmatically.
6. Be conscious of the input and output formats
By using CrewAI, we can specify the task’s expected output to conform to a Pydantic model or JSON. This is very useful, especially if we want to access the output data later.
class Blog(BaseModel):
title: str
content: str
class Captions(BaseModel):
instagram: str
twitter: str
linkedin: str
# The task's output will follow the Blog model
task1 = Task(
description=(
"""Create a blog title and content on a given topic.
Make sure the content is under 200 words."""
),
expected_output="A compelling blog title and well-written content.",
agent=blog_agent,
output_pydantic=Blog,
)
# The task output will be a JSON object that follows the Captions model
task2 = Task(
description=(
"""Generate three engaging social media post captions based on
a given topic. Ensure each caption is under 50 words and tailored
for different platforms (Instagram, Twitter, and LinkedIn)."""
),
expected_output="Three concise and platform-specific social media captions.",
agent=social_media_agent,
output_pydantic=Captions,
)
While writing a custom tool, we can make it return the output as a dictionary with meaningful keys so that it is easier for the agent to understand when compared to unstructured strings.
7. While debugging, try to understand what the agent’s thought process is
When we enable the Verbose mode, the logs are more detailed and contain information about the agent’s thought process and actions. Sometimes, we can figure out what to optimize just by reading the logs 📝. Tweaking the task description and agent’s goal would become easier, as now we know exactly what to change.
As a part of debugging, we can also make the agent explain why it made a certain decision.
class Result(BaseModel):
name:str
should_we_hire:bool
additional_comments:str
For example, here is what I did for a resume screening and candidate selection problem. I designed the agent to return results as a Result
object. The Result
model included an additional_comments
field, where the agent provided reasoning on whether to proceed with a candidate.
{
"name": "XYZ",
"should_we_hire": true,
"additional_comments": "Upon analyzing the candidate's resume
and comparing it with the job description, it is evident that
the candidate's skill set aligns closely with the technical
requirements outlined in the job description. With 5 years
of work experience in relevant roles and proficiency in the
required front-end and back-end technologies, as well as
experience with frameworks like React, Angular, Vue, and
Express, the candidate is well-suited for the position.
Therefore, based on the skill set evaluation and work
experience alignment, I recommend hiring the candidate."
}
On seeing the output, we can infer that the agent has understood that it has to cross-check with the job description and choose a candidate based on factors such as skills and experience.
8. Provide relevant examples
While explaining complicated steps that have to be performed to an agent, giving an example might help the agent understand its function better. This is actually a prompting technique called Few-shot prompting.
9. Use guard rails
Guard rails ensure that AI is not being misused and prevents it from making biased decisions and hallucinating. We can provide guard rails in the agent’s goal section to ensure that the responses follow a certain standard.
Some examples of guard rails are as follows:
Ensure the language is inclusive and respectful of all cultures
Ensure the information is accurate and cite reliable sources when possible.
Provide data-driven recommendations
10. Focus on the format of the prompt provided to the agent
When the prompt is vague and lacks clarity, the agent may hallucinate as it lacks sufficient information to make an informed decision. Hence, it is very important to ensure that the role, goal and backstory overall contain sufficient information about:
What the agent should do
Output format and length
Constraints
Context
The role, goal and backstory shape how the agent understands its purpose. When they are precise and well aligned, we get better results. Experimenting with various formats can help in determining which works the best.
Conclusion
In this blog, we have seen ten steps you can follow to improve the results while working with CrewAI.
In a nutshell 🥜,
Model agents in a way that they are specialized in doing one task each
Structure input and output data in a way that is easy for the agents to follow
Make the agent focus on decision-making and handle simpler tasks programmatically, if possible
For debugging, follow the logs and make the agent explain its choice of decisions to understand why the agent is doing something